Sheng Li
Monday, October 22nd, 2018
Adversarial Training for Sequential Data
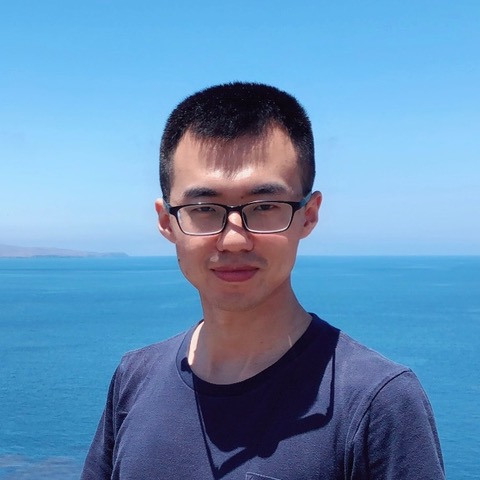
Existing approaches on sequential modeling mostly serve as ‘black-box’ methods and are limited in their ability to interpret the results. Besides, these sophisticated learning models are known to be susceptible to deliberate adversarial attacks. While there exist several defense strategies against adversarial attacks on static data, these approaches do not take into account the structural property of sequential data. To close this gap, we develop a new learning framework based on adversarial training to improve the robustness to sequence classification. Specifically, it answers the question of When and How to perturb the data when applying the adversarial training. I will demonstrate the effectiveness of the proposed method in a diversity of real-world datasets, such as e-Commerce, natural language processing and remote sensing. In addition, I will briefly introduce my recent deep learning projects on action recognition and answer selection.
Sheng Li is an Assistant Professor at the Department of Computer Science, University of Georgia. He received his Ph.D. degree in computer engineering from Northeastern University, Boston, MA in 2017, and worked as a research scientist at Adobe Research, San Jose, CA from 2017 to 2018. He has published over 65 papers at leading conferences and journals, and has received three best paper awards or nominations at SDM 2014, IEEE ICME 2014, and IEEE FG 2013. He serves as Associate Editor of IEEE Computational Intelligence Magazine, Neurcomputing, IET Image Processing, and Journal of Electronic Imaging. He also serves as SPC member for AAAI, and PC member for NIPS, IJCAI, KDD, ICLR, etc. His research interests include robust machine learning, representation learning, visual intelligence and causal inference.